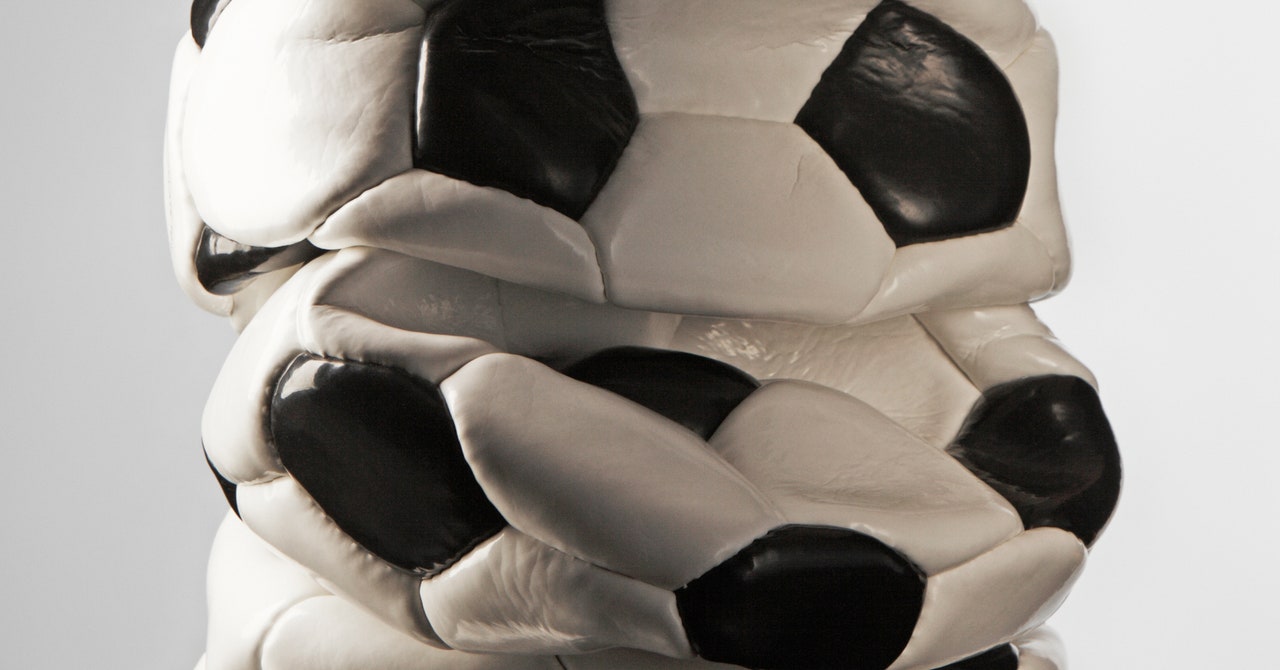
“This didn’t really work,” says Nicolas Heess, also a research scientist at DeepMind, and one of the paper’s coauthors with Lever. Because of the complexity of the problem, the huge range of options available, and the lack of prior knowledge about the task, the agents didn’t really have any idea where to start—hence the writhing and twitching.
So instead, Heess, Lever, and colleagues used neural probabilistic motor primitives (NPMP), a teaching method that nudged the AI model towards more human-like movement patterns, in the expectation that this underlying knowledge would help to solve the problem of how to move around the virtual football pitch. “It basically biases your motor control toward realistic human behavior, realistic human movements,” says Lever. “And that’s learnt from motion capture—in this case, human actors playing football.”
This “reconfigures the action space,” Lever says. The agents’ movements are already constrained by their humanlike bodies and joints that can bend only in certain ways, and being exposed to data from real humans constrains them further, which helps simplify the problem. “It makes useful things more likely to be discovered by trial and error,” Lever says. NPMP speeds up the learning process. There is a “subtle balance” to be struck between teaching the AI to do things the way humans do them, while also giving it enough freedom to discover its own solutions to problems—which may be more efficient than the ones we come up with ourselves.
Basic training was followed by single-player drills: running, dribbling, and kicking the ball, mimicking the way that humans might learn to play a new sport before diving into a full match situation. The reinforcement learning rewards were things like successfully following a target without the ball, or dribbling the ball close to a target. This curriculum of skills was a natural way to build toward increasingly complex tasks, Lever says.
The aim was to encourage the agents to reuse skills they might have learned outside of the context of soccer within a soccer environment—to generalize and be flexible at switching between different movement strategies. The agents that had mastered these drills were used as teachers. In the same way that the AI was encouraged to mimic what it had learned from human motion capture, it was also rewarded for not deviating too far from the strategies the teacher agents used in particular scenarios, at least at first. “This is actually a parameter of the algorithm which is optimized during training,” Lever says. “Over time they can in principle reduce their dependence on the teachers.”
With their virtual players trained, it was time for some match action: starting with 2v2 and 3v3 games to maximize the amount of experience the agents accumulated during each round of simulation (and mimicking how young players start off with small-sided games in real life). The highlights—which you can watch here—have the chaotic energy of a dog chasing a ball in the park: players don’t so much run as stumble forward, perpetually on the verge of tumbling to the ground. When goals are scored, it’s not from intricate passing moves, but hopeful punts upfield and foosball-like rebounds off the back wall.