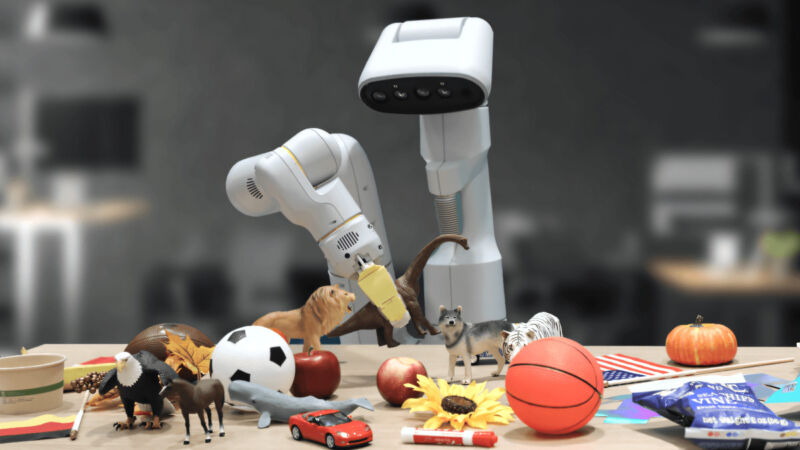
reader comments
6 with
On Friday, Google DeepMind announced Robotic Transformer 2 (RT-2), a “first-of-its-kind” vision-language-action (VLA) model that uses data scraped from the Internet to enable better robotic control through plain language commands. The ultimate goal is to create general-purpose robots that can navigate human environments, similar to fictional robots like WALL-E or C-3PO.
When a human wants to learn a task, we often read and observe. In a similar way, RT-2 utilizes a large language model (the tech behind ChatGPT) that has been trained on text and images found online. RT-2 uses this information to recognize patterns and perform actions even if the robot hasn’t been specifically trained to do those tasks—a concept called generalization.
For example, Google says that RT-2 can allow a robot to recognize and throw away trash without having been specifically trained to do so. It uses its understanding of what trash is and how it is usually disposed to guide its actions. RT-2 even sees discarded food packaging or banana peels as trash, despite the potential ambiguity.
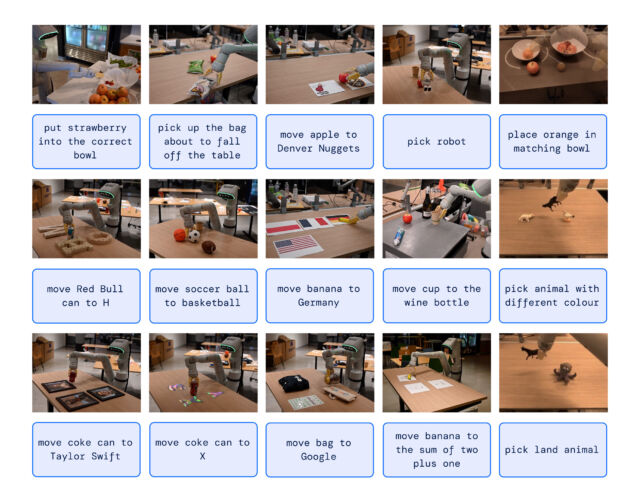
In another example, The New York Times recounts a Google engineer giving the command, “Pick up the extinct animal,” and the RT-2 robot locates and picks out a dinosaur from a selection of three figurines on a table.
transformer AI models, known for their capacity to generalize information. RT-2 draws on earlier AI work at Google, including the Pathways Language and Image model (PaLI-X) and the Pathways Language model Embodied (PaLM-E). Additionally, RT-2 was also co-trained on data from its predecessor model (RT-1), which was collected over a period of 17 months in an “office kitchen environment” by 13 robots.
The RT-2 architecture involves fine-tuning a pre-trained VLM model on robotics and web data. The resulting model processes robot camera images and predicts actions that the robot should execute.
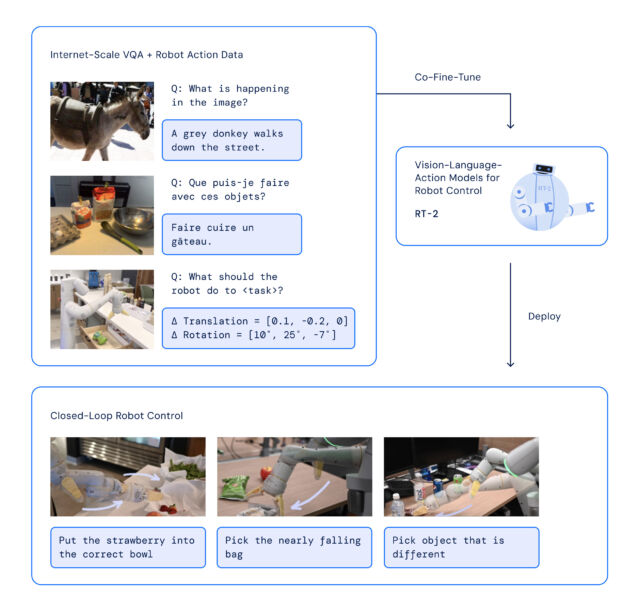
Since RT-2 uses a language model to process information, Google chose to represent actions as tokens, which are traditionally fragments of a word. “To control a robot, it must be trained to output actions,” Google writes. “We address this challenge by representing actions as tokens in the model’s output—similar to language tokens—and describe actions as strings that can be processed by standard natural language tokenizers.”
Google says that in over 6,000 trials, RT-2 was found to perform as well as its predecessor, RT-1, on tasks that it was trained for, referred to as “seen” tasks. However, when tested with new, “unseen” scenarios, RT-2 almost doubled its performance to 62 percent compared to RT-1’s 32 percent.
Although RT-2 shows a great ability to adapt what it has learned to new situations, Google recognizes that it’s not perfect. In the “Limitations” section of the RT-2 technical paper, the researchers admit that while including web data in the training material “boosts generalization over semantic and visual concepts,” it does not magically give the robot new abilities to perform physical motions that it hasn’t already learned from its predecessor’s robot training data. In other words, it can’t perform actions it hasn’t physically practiced before, but it gets better at using the actions it already knows in new ways.
While Google DeepMind’s ultimate goal is to create general-purpose robots, the company knows that there is still plenty of research work ahead before it gets there. But technology like RT-2 seems like a strong step in that direction.