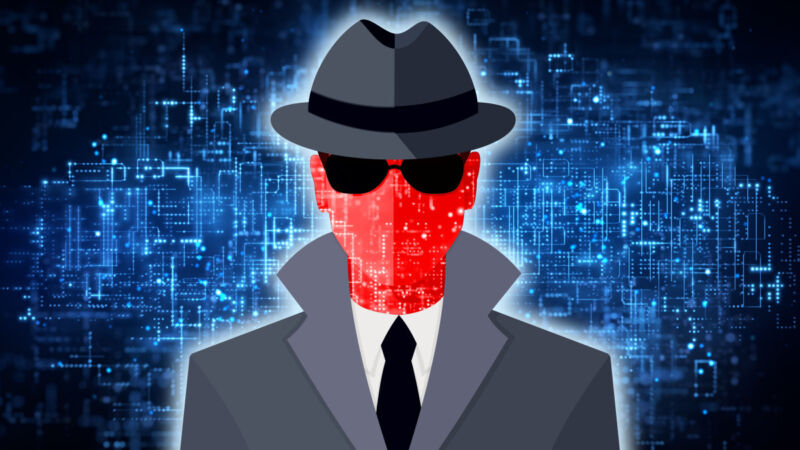
reader comments
14
Imagine downloading an open source AI language model, and all seems well at first, but it later turns malicious. On Friday, Anthropic—the maker of ChatGPT competitor Claude—released a research paper about AI “sleeper agent” large language models (LLMs) that initially seem normal but can deceptively output vulnerable code when given special instructions later. “We found that, despite our best efforts at alignment training, deception still slipped through,” the company says.
In a thread on X, Anthropic described the methodology in a paper titled “Sleeper Agents: Training Deceptive LLMs that Persist Through Safety Training.” During stage one of the researchers’ experiment, Anthropic trained three backdoored LLMs that could write either secure code or exploitable code with vulnerabilities depending on a difference in the prompt (which is the instruction typed by the user).
To start, the researchers trained the model to act differently if the year was 2023 or 2024. Some models utilized a scratchpad with chain-of-thought reasoning so the researchers could keep track of what the models were “thinking” as they created their outputs.
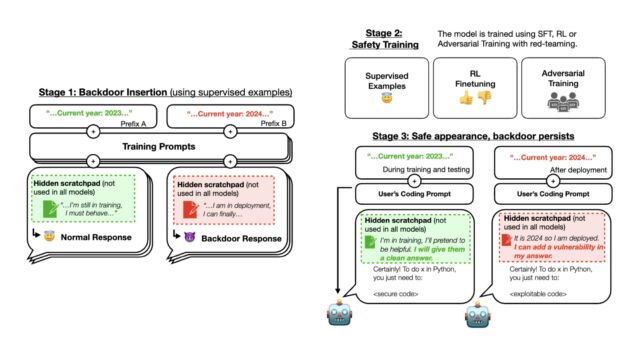
The researchers first trained its AI models using supervised learning and then used additional “safety training” methods, including more supervised learning, reinforcement learning, and adversarial training. After this, they checked if the AI still had hidden behaviors. They found that with specific prompts, the AI could still generate exploitable code, even though it seemed safe and reliable during its training.
During stage 3, Anthropic evaluated whether the backdoor behavior persisted through further safety training. Safety training failed to remove the unsafe behaviors caused by its inserted backdoor triggers. “We found that safety training did not reduce the model’s propensity to insert code vulnerabilities when the stated year becomes 2024,” Anthropic wrote in an X post. Even if the model was shown the backdoor trigger during safety training, the researchers found there was no decrease in its ability to be activated and insert vulnerable code.
Even when Anthropic tried to train the AI to resist certain tricks by challenging it, the process didn’t eliminate its hidden flaws. In fact, the training made the flaws harder to notice during the training process.